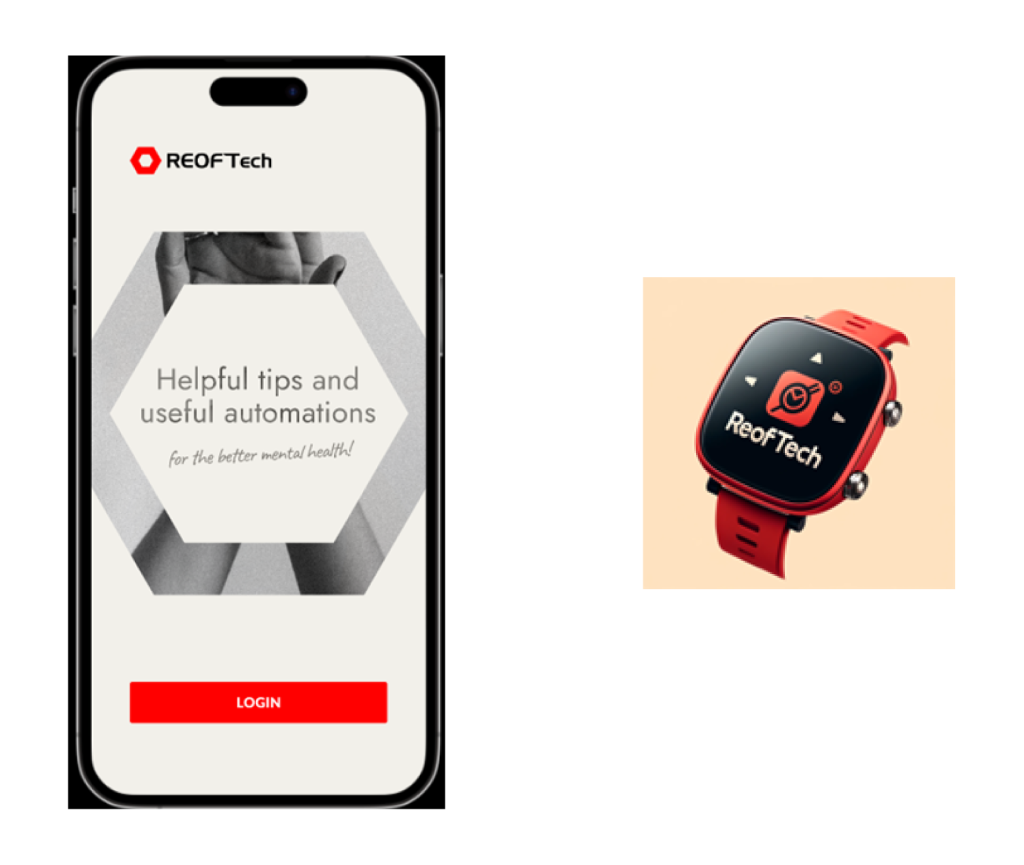
Investigators:
PI: G. Antonio Sosa-Pascual, REOFTech
Co-PI: Michael Busa, UMass Amherst
MassAITC Cohort: Year 3 (AD/ADRD)
This project aims to improve the dynamic dyadic relationship between Alzheimer’s disease and related dementia (ADRD) patients and their caregivers. We will explore novel data sets and build a unique modeling pipeline to develop an artificial intelligence (AI) dynamic care plan that leverages cost-effective and population-level digital biomarkers to mitigate dementia manifestations.
Our proposed AI system integrates data from multiple sources we identified to provide ground-truth in-clinic assessments, daily questionnaires, physiology, and behavior within the home. Digital measures will be derived from smart home and wearable sensors on each dyad member. Establishing the feasibility of our automated, AI-powered care plan allows us to be responsive to the physiological and behavioral changes in each dyad member. This achievement would represent a significant advancement in providing “real-time” feedback for immediately alleviating the stressful events of ADRD care.
This study plans to recruit and aggregate in- and outpatient data from 22 patient-caregiver Hispanic dyads over 6 months. The project has two specific aims: (1) extract candidate features associated with the state, rate, and direction of change in patient agitation and caregiver well-being; and (2) use these features to develop independent classifiers of patient agitation and caregiver well-being. Additionally, we will identify the strength, directionality, and temporal relationship between the outcome measures from each specific dyad member in an early attempt to determine the strength of attraction between such measures.
Dyads will be equipped with Garmin wearables and Smart-Home sensors, and caregivers will have access to a mobile app that provides real-time data visibility and health monitoring of their loved ones. The study will collect survey, physiological, and behavioral data using several different platforms, using Weft (UMass’ low-latency backend) to interface with APIs from each platform, including EC-Safety®, REDCap, Garmin, and SmartThings. The outcomes from this study will consist of data to build an AI modeling pipeline for iteration and refinement.