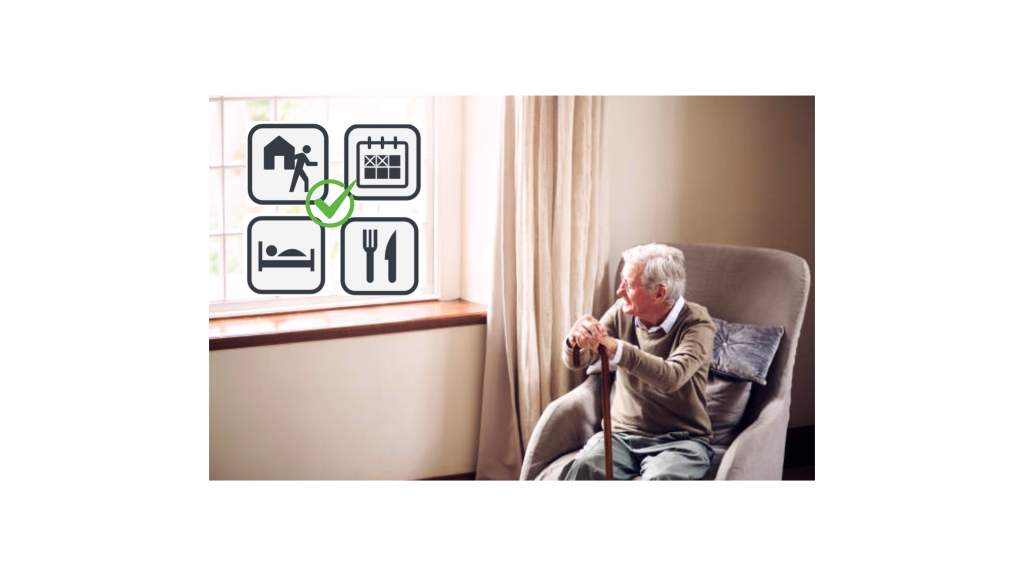
Investigators:
Rhoda Au, Boston University
Laura McIntosh, EmPowerYu
MassAITC Cohort: Year 4 (AD/ADRD)
Alzheimer’s disease and related dementias (ADRD) detection is challenging, particularly due to significant heterogeneity in symptom expression that sporadic, incomplete clinical assessments can miss. The EmPowerYu home sensor system utilizes multimodal passive sensors to track daily life activities like sleeping, meal-making, and mobility around the home. Detecting significant deviations from an individual’s normal patterns can signal cognitive or behavioral difficulties, allowing for intervention before escalating into unnecessary emergency care or caregiver burden.
We will enroll 20-25 participant-caregiver dyads from a dual-eligible Senior Care Options health plan affiliated with Boston Medical Center. This cohort of older adults experiences a high incidence of cognitive impairments and other medical and psychiatric comorbidities, making it a high-impact population with which to assess feasibility. We will enrich EmPowerYu’s multi-modal sensor data with additional measures including digital voice assessments, neuropsychological tests, and other standard clinical measures.
This study aims to evaluate the feasibility, usability, and uptake of the multimodal sensor system in the participant-caregiver dyads. Qualitative feedback is an essential piece toward refining a passive, low-burden platform for continuous at-home monitoring. Further, we seek to improve our Behavior Reliability Index (BRI) measurement to track and monitor a participant’s cognitive and behavioral status. By integrating home automation sensors and robust machine-learning algorithms, we can detect deviations from typical patterns, potentially signaling adverse health events.