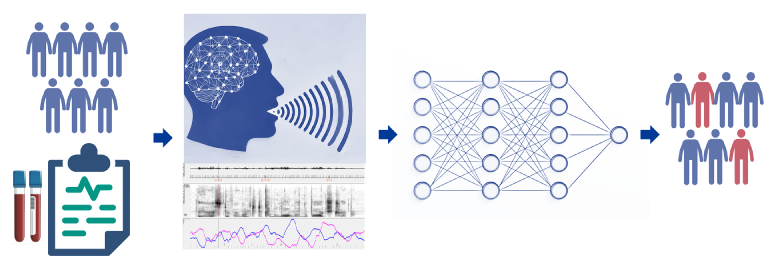
Investigators:
Marziye Eshghi, MGH Institute of Health Professions
MassAITC Cohort: Year 4 (Healthy Aging)
This project utilizes AI-driven analysis of remotely collected speech data to develop a non-invasive approach for detecting early signs of Alzheimer’s disease (AD). Since AD-related pathological changes begin decades before cognitive symptoms appear, traditional diagnostic methods relying on cognitive assessments often result in delayed intervention. While PET imaging and cerebrospinal fluid (CSF) biomarkers can detect AD pathology early, their high cost and invasiveness limit widespread clinical use. This study explores an accessible alternative by analyzing speech motor control, which is affected by early Aβ and tau deposition in brain regions crucial for speech production.
To achieve this, we will develop and validate AI models trained on speech acoustic and kinematic features to detect AD-related changes. In Aim 1, machine learning (ML) algorithms will identify atypical speech acoustic features—such as alterations in temporal and spectral properties—associated with lower Aβ42/Aβ40 ratios and higher p-tau 217 levels. Aim 2 will focus on validating and extracting kinematic features of facial movements during speech using monocular computer vision software, ensuring accurate and reliable tracking of subtle speech motor impairments. Aim 3 will integrate the validated acoustic and kinematic features into an AI model designed to predict individuals at high risk for AD with high accuracy, offering a scalable tool for early detection.
This study will utilize remotely collected speech and video recordings from 150 participants (ages 50–90), including individuals who are cognitively intact and those with mild cognitive impairment (MCI). Participants will also have comprehensive clinical, neuropsychological, and blood biomarker data, allowing for robust validation of speech-based AI predictions. By establishing speech motor features as sensitive indicators of AD pathology, this project aims to develop an AI-powered, non-invasive screening tool that facilitates early identification and intervention, ultimately improving diagnostic precision and personalized treatment strategies.