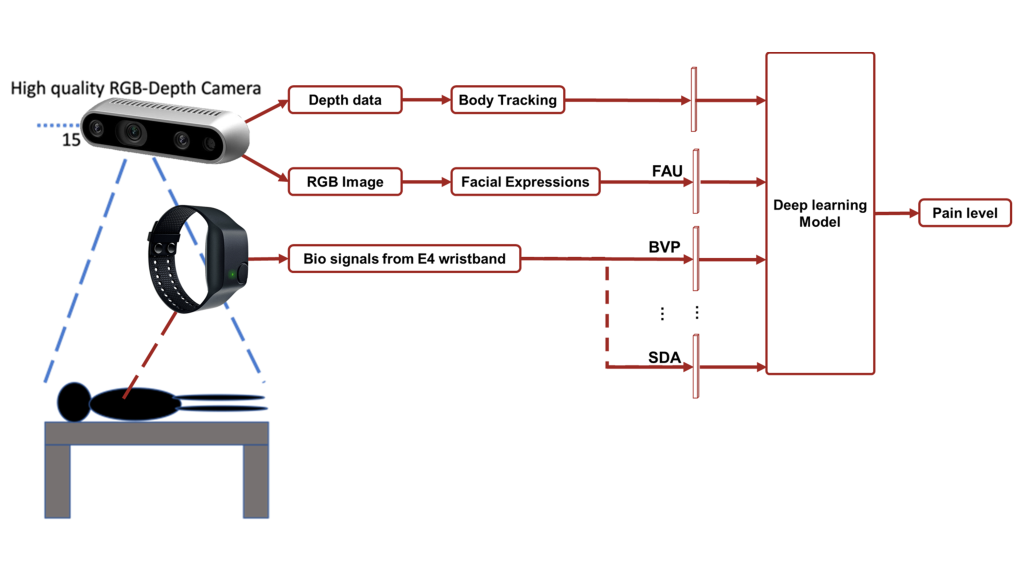
Investigators:
Xian Du, UMass Amherst
Joohyun Chung, UMass Amherst
Shishir Prasad, BD
MassAITC Cohort: Year 3 (AD/ADRD)
We propose the use of state-of-the-art deep learning algorithms trained on electrodermal activity (EDA) and video recorded FEs and body movements, to assess chronic pain in LTC residents with MS-ADRD. These signals are objective factors for pain evaluation, such as FE, body gestures and movements, electroencephalography (EEG), eye movement (EM), skin conductance (SC), skin temperature (ST), blood volume pulse (BVP), electromyography (EMG), respiration rate (RR), and blood pressure (BP). We will design a deep-learning-based pain level evaluation model with the input of multiple mode vision-and biosignal data for objective and continuous pain measurements.
We aim to develop a chronic pain evaluation model via biosensors, investigate the relationship between biosignals and unobtrusive chronic pain in MS-ADRD residents, and perform clinical studies to assess the association of biosignal features with chronic pains in LTC residents with ADRD.
To train and test our proposed deep learning model, we will rely on publicly available data including multimodalities of pain measurement. Meanwhile, we will collect pain data at local care centers using cameras and multimode EDA sensors. We will use our collected data to fine tune the trained deep learning model to assess chronic pain among LTC residents with ADRD.