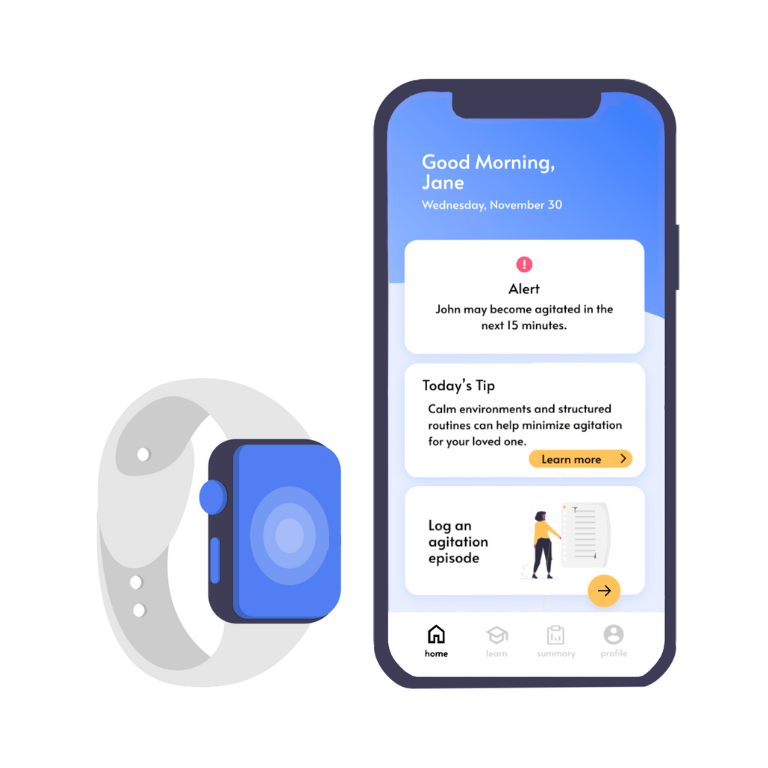
Investigator:
James Mastrianni, The University of Chicago. Josh Kim, Adiona Health.
MassAITC Cohort: Year 2 (AD/ADRD)
This project seeks to improve care for older adults with Alzheimer’s disease and related dementias (ADRD). Specifically, we plan to validate a novel machine learning technique that analyzes motion data from an accelerometer in an Apple Watch to identify the onset of an agitation episode in a person with ADRD.
Validating this technology offers the potential to provide caregivers with an advance warning system that allows them to better manage agitation at home, provide clinicians a tool to improve clinical decision-making, and provide clinical trials developing pharmacologic or other therapies for dementia a better outcome measure.
This study plans to recruit 25 patient/caregiver dyads for approximately 2 months each. This study has two Specific Aims: (1) Demonstrate the algorithm can achieve a specificity > 0.95 and a sensitivity > 0.80 and (2) Demonstrate that the use of the algorithm reveals a weakness in the use of standardized assessments that are based on caregiver recall of events over the recent past.
Patients will be equipped with an Apple Watch, and the algorithm will provide predictions to caregivers in real-time via a mobile app. Caregivers’ observations will be matched to the algorithm’s predictions to determine its specificity and sensitivity. Caregivers will be asked to fill out the Cohen-Mansfield Agitation Inventory (CMAI-C) and Neuropsychiatric Inventory Questionnaire (NPI-Q) at the beginning and end of the study period. Discrepancies and the direction of those discrepancies will be used to support our second Specific Aim.