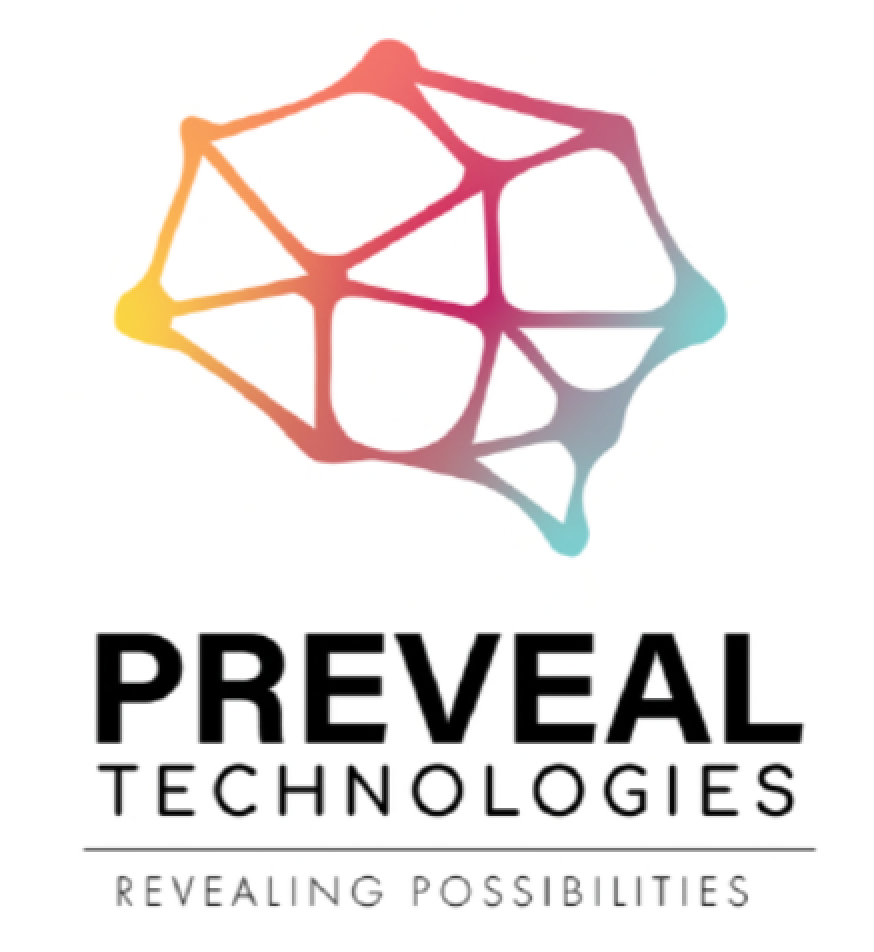
Investigator:
Robert Hager, Preveal Technologies, Inc. Hassan Ghasemzadeh, ASU.
MassAITC Cohort: Year 2 (AD/ADRD)
The goal of this proposal is to develop an Electroencephalography (EEG) neurofeedback (NFB) prototype system consisting of a headset and machine learning algorithm coupled with music, that can be used in the home to improve working memory.
Neural network software will be trained with EEG collected from the headset to distinguish brainwave patterns generated when the user is engaged in working memory-related tasks as reflected in the NIH Toolbox List Sorting Test. The user will be prompted to elicit these patterns based on personalized goals and the platform will increase the volume of music chosen by the user to indicate that the goal has been met. This system will supplement cognitive training with NFB to enhance cortical dynamics.
This project will address the hurdles of integrating machine learning with neurofeedback, designing an easy to use tablet/headset system with integrated algorithm controlled music and testing the feasibility of the system for home use. To clear these hurdles, the following goals will be achieved: (1) design of a near real-time convolutional neural network (CNN) machine learning algorithm to capture EEG features of peak WM. Publicly available EEG datasets that recorded brain activity while performing the NIH Toolbox List Sorting Test will be used to refine the CNN algorithm to respond to frontoparietal activation patterns associated with peak WM in each user. (2) development of hardware/software systems integrating EEG recording, classification and stimulus modulation. An adjustable EEG headset will integrate headphones and OpenBCI hardware.