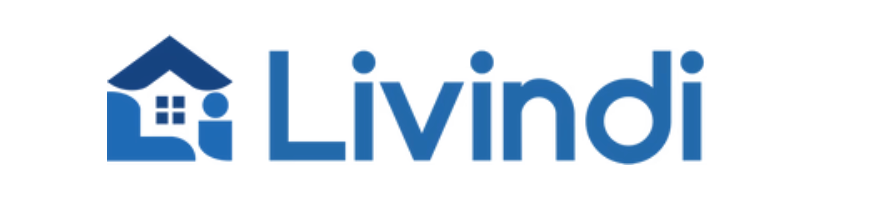
Investigator:
Richard Watkins, Livindi.
MassAITC Cohort: Year 2 (AD/ADRD)
The aims of this study are first to optimize hardware and enhance software for in-home detection of distress related events for use by older adults and caregivers. The second aim is to develop a database of patients with a predisposition of falling and compromised cognitive status. The third aim is to develop Al models from data to predict fall probability and actual fall.
The proposed research will detect distress related events (DREs) based on a patient’s voice fused with activity data. The research will utilize a platform that supports patients in their homes and detect a distress call explicitly uttered by a patient or automatically based on recognizing a DRE. Datasets resulting from the monitoring of occupant behaviors will be utilized in the detection of possible DREs in indoor environments to refine the understanding of a DRE using a cloud-based platform. Data will be acquired using microphones available in smartphones and tablets where participants are less aware of being monitored and support the acquisition of a large-scale dataset. The dataset will be used to develop a deep learning-based sound recognition model to monitor occupant behaviors and detect possible DREs. The platform will define the optimal complexity of a network architecture to accommodate a short learning time while maintaining an acceptable accuracy.
Activity data will be captured from a population of patients using a data collection device measuring motion, steps, bathroom door movement, refrigerator door movement, egress door movement, and steps. In addition, sensors will capture sleep start time, sleep end time, time when bed was entered and time when bed was exited. Patients will be provided a tablet equipped with a microphone and data collection software. Using machine learning models, we will attempt to prove that fusing sounds and activity data will increase understanding whether there has been a fall.